On May 29th and 31st, Serban Cristian Tudosie (Fig. 1) and Marcos Obando (Fig.2), candidates of the CONcISE doctoral training network, gave two special lectures on Machine Learning and Adaptive Systems. Hosted at Politecnico di Milano, the lectures introduced elements of machine learning and provided insightful material on the possible applications of AI in biomedical imaging. These lectures allowed members from different scientific backgrounds to understand each other better and promote collaboration.
Now, CONcISE members are looking together for new ways to reduce the data that imaging instruments acquire. They do this by using AI techniques, such as encoder-decoder architectures, a topic that gained much attention during the first lecture. Such methods are powerful for data compression and real-time de compression. But there is more: the members are now inserting physics directly into the AI architecture to gain more performance on real sys-
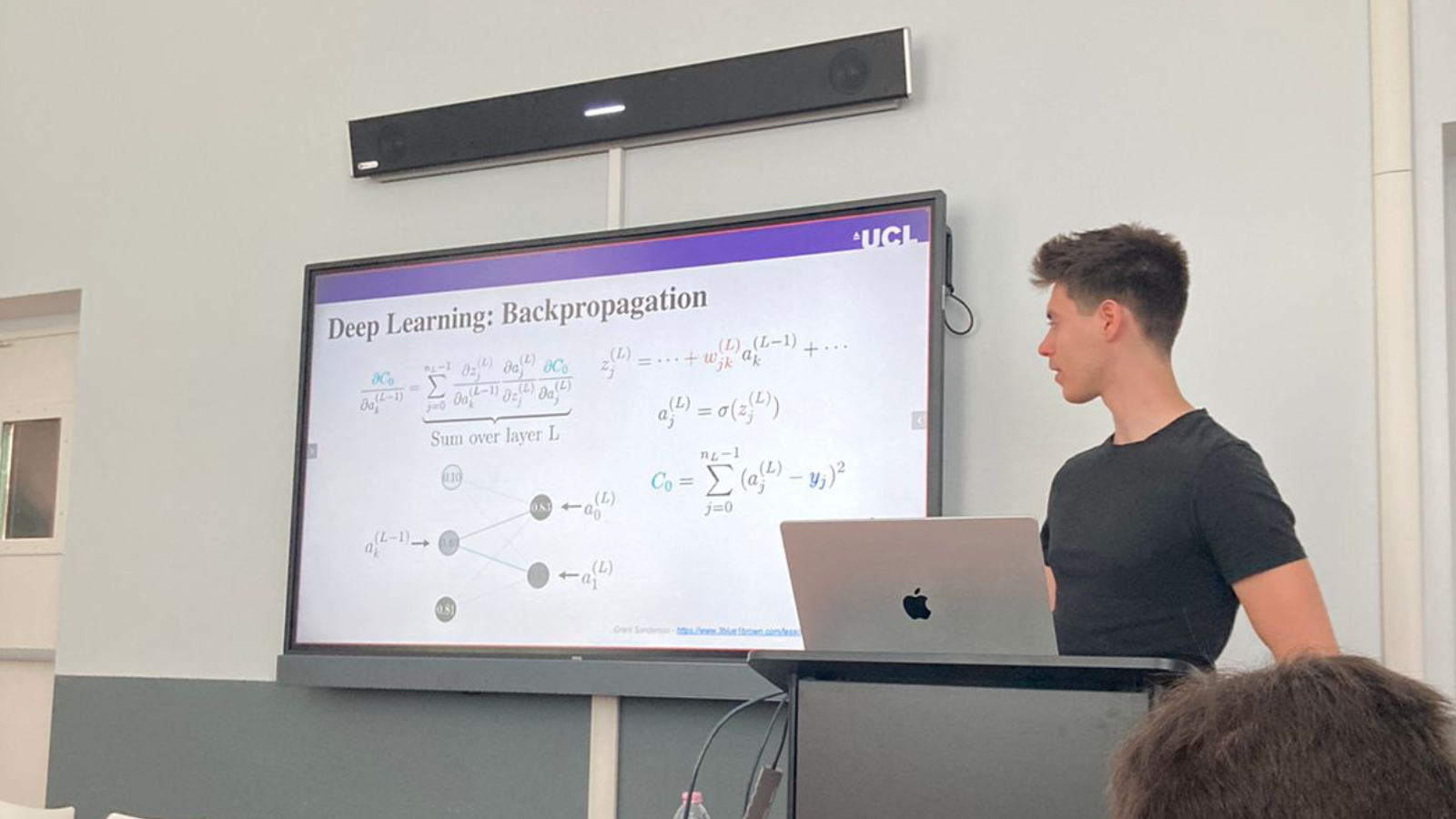
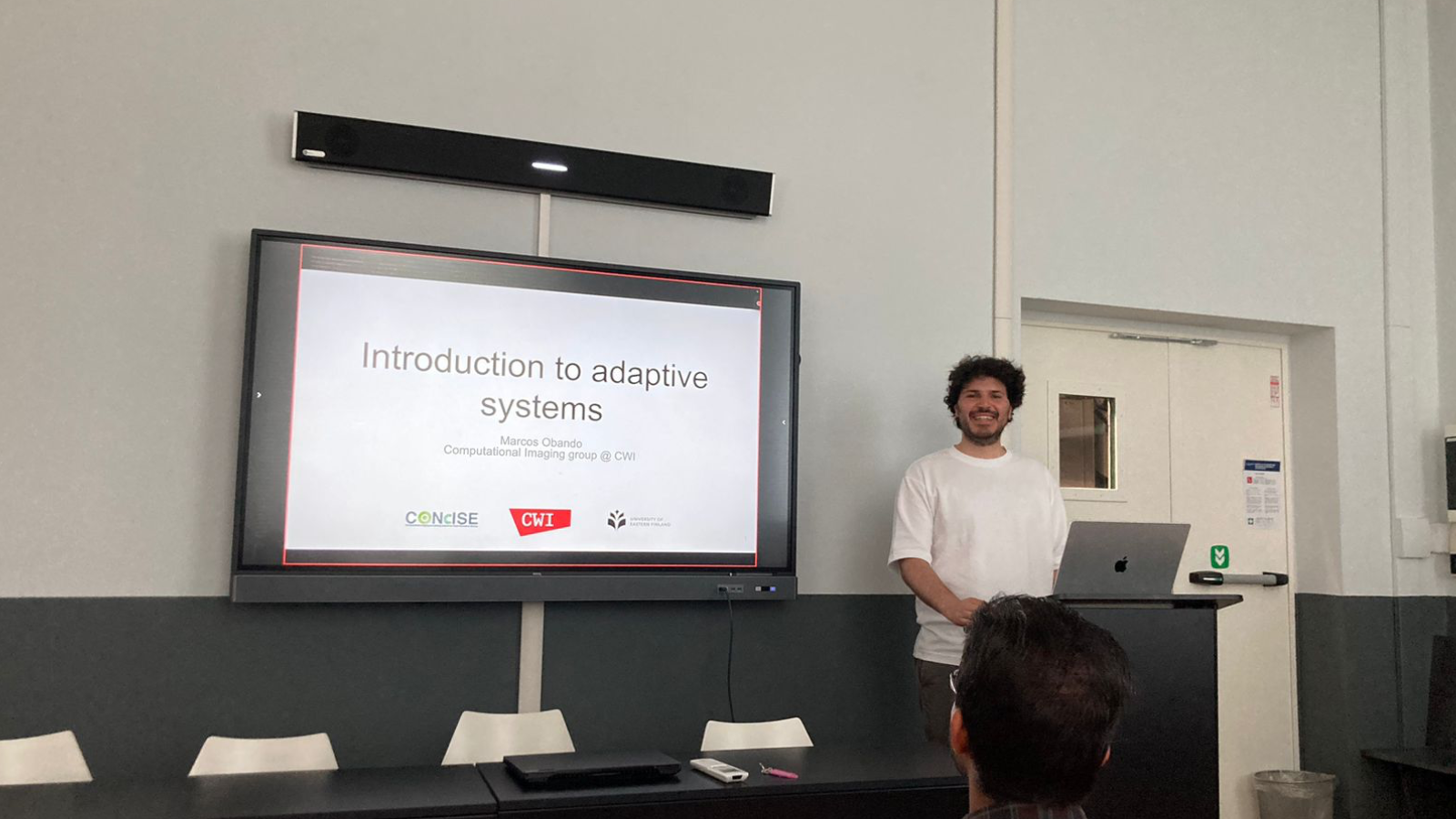
-tems such as microscopes and endoscopes. Thus, physics-informed AI results in a crucial binding for novel developments in biomedical imaging devices where many limitations of speed, noise, and incomplete measurements arise.
Another relevant discussion surged around adaptive methods for enhancing imaging devices. Instead of repeatedly acquiring the same images that lack new information and result in massive storage, adaptive sensing relies on learning from past measurements, allowing optimal compression. With reinforcement learning, candidates approach this using agents (computational programs that can make decisions based on situations they have previously faced). However, combining high-resolution and highly informative measurements is a complex problem in imaging devices, but novel hardware (video cards) and high-performance computing (supercomputers) allow this research to happen at an accelerated pace.